Earlier this month, The Atlantic CEO Nicholas Thompson posted a short video on LinkedIn (he does them every day – and they’re super interesting) about this paper, saying that Large Language Models (LLMs) are “increasing the number of people employed in translation.” That’s not exactly what the video said and it is not what the research paper says, but, hey, it made me click on it.
LLMs, and generative AI models use algorithms trained on a lot of text (A LOT of text) to recognize complex patterns. The more text and greater computing power they employ, the better the model performs. At the foundational level, they learn to respond to user requests with relevant, in-context content written in human language – the kind of words and syntax people use during ordinary conversation.
The paper said that LLMs can speed up and even improve translations, particularly for less experienced translators. The researcher looked at training compute, which is the amount of computational power required to train an LLM. The more training compute an LLM has, the faster and better a translator can work. In theory, this could lead to translators working more quickly and thus earning more money for the same number of words.
Clearly, neither Nick Thompson nor the paper’s author work in translation. Post machine translation editing fees are often much lower than fully human translation ones. So, I think it’s a leap to say that more people are making money from translating, but it is reasonable to conclude that translators can work more efficiently.
However, I think the research could yield interesting findings about the quality of LLMs and translations in lower-resource languages. The researcher used translators working from English into Arabic, Hindi, and Spanish. It’s well documented that LLMs currently work best in English and other languages that have a large digital presence. Spanish also has a large digital presence, but Arabic and Hindi – not so much.
LLMs may make translation slower in some cases
The study focused on strengthening the argument that the more resources an LLM has in terms of its training compute, the faster and better the translation is. The data supports this hypothesis. It’s hardly surprising.
The researcher didn’t provide the data on whether or not LLMs helped or hindered translation in the first place. Machine translation (a different application of AI) can speed up translation in languages for which lots of digital data is available, and it’s widely assumed that LLMs are the same, if not better. There has been little (if any?) analysis if the same is true for low-digital-resource languages. And this is the first study I’ve seen where the data suggests that it might take longer to translate content using an LLM for Arabic.
And that’s interesting because it supports the idea of how critical digital language data is.
There are 2 big issues here:
- A recognition of the problem – and that it won’t just go away or “big tech” will just build data for low resource languages.
- An acknowledgement of the critical need of resources to develop data and tools that will help narrow the digital gap for speakers of low-resource languages.
The case for developing language technology
This is a global problem: almost half the world’s population, 3.7 billion people, do not speak a major language. It’s no coincidence that those 3.7 billion also have the lowest incomes and are the most marginalized. The problem is particularly acute in crisis situations because datasets cannot be built during a crisis and this blocks access to life-saving information.
A combination of low literacy, a lack of information in local languages, and very little digital information available means that 3.7 billion people will continue to struggle to get information unless there is a fundamental shift in access. While the other half of the world’s population can get information in a language they understand on their phones, computers, and tablets, the most marginalized struggle.
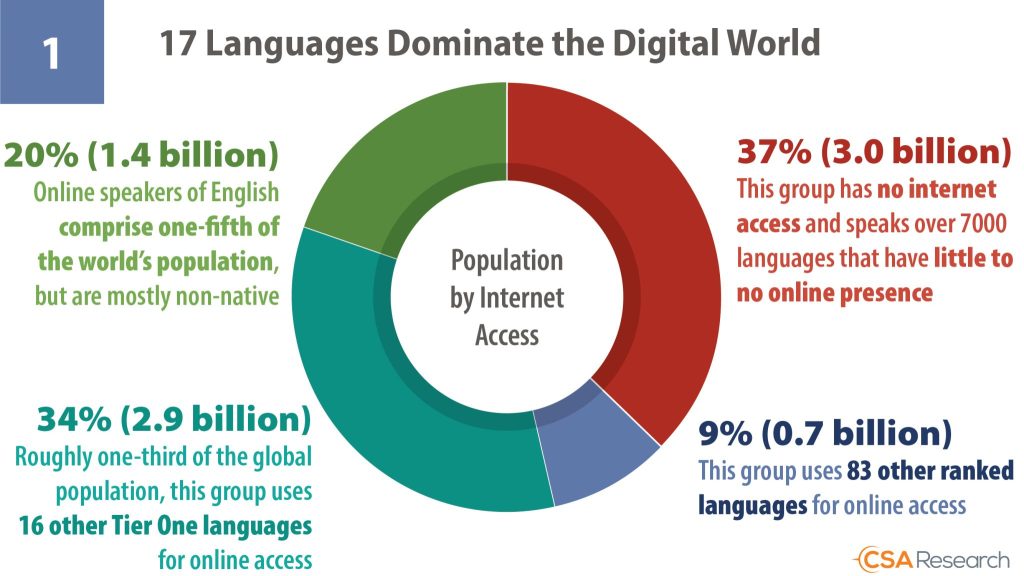
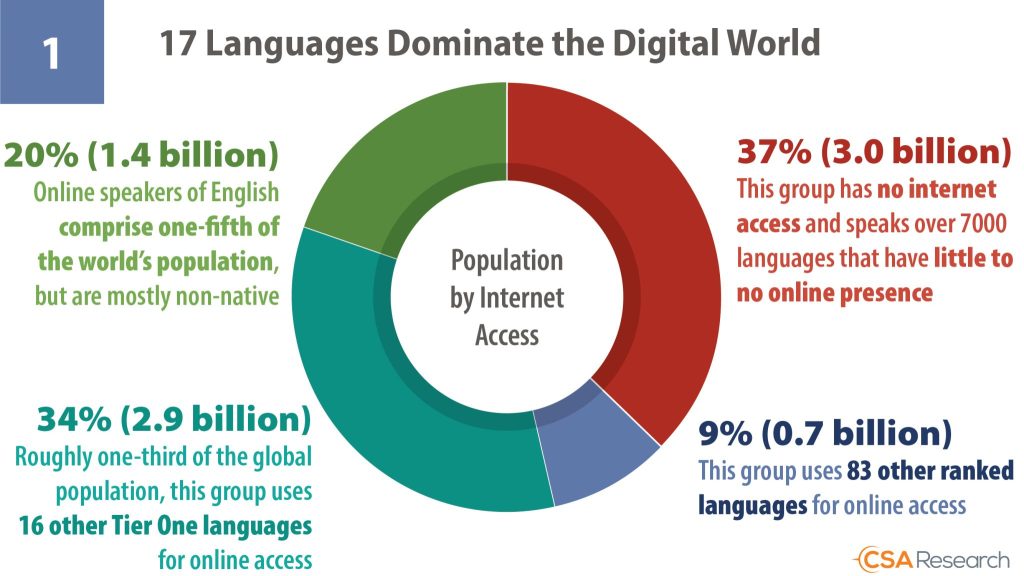
Infographic by CSA Research.
LLMs and language diversity
Language technology and large language models are evolving rapidly, yet only a fraction of the world’s 7,000 languages are meaningfully online.
The development of language technology in low.-resource languages is not increasing quickly enough to bridge the gap, particularly speech and voice technology. In my estimation, around 45 languages have enough training data. Efforts are underway to create more inclusive LLMs that encompass the world’s linguistic diversity. Governments and organizations are creating datasets and models for underrepresented languages. We work with initiatives in India, South Africa, and the UAE, in addition to the efforts of other organizations.
These efforts are a great start, but in our experience, flipping the script on this will require adequate resources, consistent engagement and collaboration for a cohesive and fruitful approach that allows for scale-up.
A call for inclusive language technology
As technology progresses and language models evolve rapidly, access to language tech is improving for some but creating a growing gap for others. For LLMs to truly benefit everyone, governments and donors need to invest in developing digital data. And LLMs need to be trained on more diverse data. This involves creating resources and datasets for underrepresented languages, involving local communities and researchers, and ensuring that AI development is inclusive and equitable. The future of LLMs may be bright, but it’s crucial to ensure that these powerful tools are developed responsibly and inclusively, benefiting the most marginalized.
I appreciate this article and find it insightful, but I wonder if we should invest more energy in post-translation evaluations. Many translations generated by LLMs, particularly for low-resource and gender-neutral languages like Luganda or Swahili, are often not fluent or adequate. Translating from these languages is even more challenging. If we want to improve LLMs’ natural language capabilities, focusing on refining the post-translation process is crucial. This is especially important as we address the broader issue of language inclusion in AI, ensuring that underrepresented languages receive the attention they need for equitable progress.