Language Use Data Platform
Helping you make data-driven decisions about language and communication in your programs
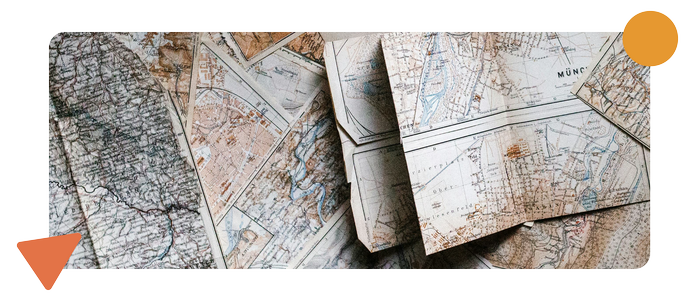
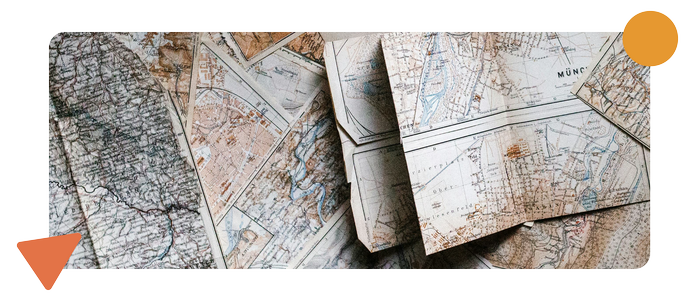
Explore language use data
Explore, visualize and download data on language use on our interactive platform. We currently cover over 30 countries and 1,000 languages with more being added all the time.
Contribute
Help us close the gap on language use data by collecting, sharing and contributing data to our platform.
Download pre-written and translated questions about language use for surveys and assessments, plus resources and advice to help you collect and use the data in your programs
Submit a dataset for inclusion in the platform
CLEAR Global’s Language Use Data Platform provides open, accessible data about the languages used across the world, including what proportion of the population speak that language.
Using public data from various reliable sources, we process, structure and assess the data to provide the most accurate and up-to-date information possible. The platform is an active system, with new data being added all the time to improve coverage and accuracy. Therefore you may find data changes on a regular basis, details of which will be posted here.
Currently the platform includes data for people’s ‘main language’ i.e. the primary language they speak. However, we will include further metrics such as secondary language and other language and communication data in the future.
You can access the data via our interactive visualizations where you can filter and display information in various ways and download versions of the visuals and datasets for use offline – see here for guidance on how to use them. You can also use our API to extract or integrate data into your own systems and analyses – see API documentation here.
Collect, use and integrate language use data in your work
Brief: The 2021 multi-sector needs assessments should collect data on the languages of affected people
Read the brief in English or French.
This brief argues that MSNAs are a critical opportunity to strengthen the evidence base for effective and accountable humanitarian response plans. It provides recommended language and communication questions, and key considerations on how to include them in MSNAs.
Five easy steps to integrate language data into humanitarian and development programs
Data on the languages of affected people is as important for meeting their needs as data on their age and gender. This tool is a quick reference guide to your options on how to use language data at different stages of planning and delivering aid programs.
Blog: Language data fills a critical gap for humanitarians
Learn how language use data informs more effective and inclusive programming
Global literacy map by gender
Why we need to collect data on the languages of crisis-affected people (PDF)
This infographic highlights the challenges we face when we fail to incorporate language data into humanitarian decision making. In order to address those challenges, we propose four key questions to include in all humanitarian data collection efforts.
View the infographic in English, Congolese Swahili, French and Lingala (Facile).
MSNA language data can help humanitarians communicate better with affected people (PDF)
This brief summarizes key language and communication findings from the 2019 Multi-Sectoral Needs Assessment in northeast Nigeria. It illustrates the potential for large-scale surveys of this kind to fill critical language and communication information gaps throughout the humanitarian sector.
Blog: When words fail: audio recording for verification in multilingual surveys
Rapid guide to localizing and translating survey tools
The words between us: How well do enumerators understand the terminology used in humanitarian surveys?
This report demonstrates that languages is not a routine consideration in survey design. It concludes that enumerators often do not understand the words they must translate in surveys.
Putting language on the map in the European refugee response
Licensing
Data is provided with a Creative Commons Attribution Share-Alike license (CC BY-SA).
Under the CC BY-SA license, you are free to share (copy and redistribute the material in any medium or format) and or adapt (remix, transform, and build upon the material) for any purpose, even commercially. The licensor cannot revoke these freedoms as long as you follow the license terms. The license terms are that you must give appropriate credit, provide a link to the license, and indicate if changes were made. You may do so in any reasonable manner, but not in any way that suggests the licensor endorses you or your use. If you remix, transform, or build upon the material, you must distribute your contributions under the same license as the original. Additionally, you may not apply legal terms or technological measures that legally restrict others from doing anything the license permits.
Methodology Notes
Data sources: Data is sourced from various reliable publicly available data sources, including census data via IPUMS, The DHS (Demographic & Health Surveys) Program, AfroBarometer and others. Data is scraped from these sources on a regular basis. More data sources will be added over time. Additionally individual datasets outside of these core sources may be sourced and included in the system providing they meet our standards and requirements. The source of any specific data point is always identified in the visualisations provided, and data via the API.
Data processing and classification: All datasets input to the system are aligned with our dynamic referencing system along two key dimensions: Languages and Geographic location. Our primary source for language classification is Glottlog and for locations UN OCHA’s Common Operational Datasets. As we build our data, our referencing system will also build to include data outside of those systems, however, it will always be aligned to those sources in the first instance.
Data scoring & review: Once aligned with these core references source data is assessed against a weighted set of criteria to determine its reliability. The criteria are:
- Timeliness: The age of the data based on when data collection occurred (or if not available, then when data was published). More recent datasets score higher, older ones lower.
- Representivity: How statistically representative the dataset is based on the sampling methodology, confidence level and margin of error. Census datasets are generally scored highest, and non-representative/indicative datasets lowest.
- Language variable quality: How good was the survey question about language used? There are a number of ways surveys ask people about the language they speak, from ‘Mother tongue’ to ‘What is the main language you use at home’. This can affect the accuracy of the data on language. Variables most closely aligned to our recommended questions are rated highest, and variables that are poorly worded or proxies for language use are rated lowest.
- Spatial granularity: At what level of geography the data is gathered. Language is generally geographically distributed therefore datasets with a greater level of geographic detail are both more useful and more accurate.
- Dataset size: How many observations are in the dataset. We preference larger datasets for better accuracy.
All datasets are scored against these criteria and given an aggregate ‘reliability score’. This score is used to determine which is the most accurate and useful dataset where we have multiple datasets for a given location. Generally the highest scoring data is published on the platform. We also review each dataset and publish only those that are sufficiently accurate and complete.
The ‘reliability score’ for any data point is shown in both the visualisations and in the API allowing you to assess the likely accuracy of the data.
Using the Interactive Vizualisations
There are two ways to view the data in our interactive vizualisations:
- Languages of Country/Province/District: This shows the languages spoken in a given location (country, province or district) including a map of the most common main language spoken in each area
- Language: This shows details about a given language, including the locations it is spoken in.
You can interact with the vizualisations as follows:
- Change the data shown by filtering for a Country, Province or District (on the Languages of Country/Province/District page) or Language Name (on the Language page)
- Change the view between a map, chart or table with the View option.
- View data at a higher or lower level of geographic detail (i.e. at country, province or district level) by clicking Drill Up or Drill Down
- Navigate to more details on a language or a location by clicking on an item in the map, chart or table and clicking on See details on…
- Use the Download and Share buttons to download data and visuals in various formats or get a shareable link to the view (Link)
- See details about the original source of the data under Sources